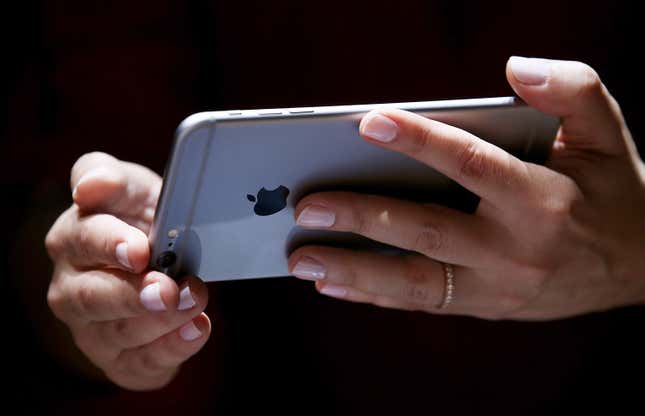
A common misconception equates the use of correct English with “talking white.” It’s a false and toxic notion, but that doesn’t necessarily mean “talking white” or “talking black” aren’t actual things. Perhaps it just has more to do with tone and pitch than it does word choice or pronunciation. How easy is it for you to tell whether a black person or a white person is speaking without looking?
According to Business Insider, a study published by the National Academy of Sciences Monday found that racial bias is a major issue with the automated systems of big tech companies.
The study focused on Amazon, Apple, Google, IBM, and Microsoft to see how accurate their voice-recognition systems are at transcribing black voices in comparison to white voices and found that the error rates for white voices were much lower.
The researchers gave the voice-recognition systems almost 20 hours of interviews to transcribe from 42 white and 73 black interviewees. The average error rate for the white interviewees was 19%, whereas for the black interviewees it was 35%.
The study mirrors other research which has revealed: AI facial recognition can become racially-biased, more frequently misidentifying people with darker skin tones. This is due to the datasets being composed predominantly of white people.
Microsoft’s system performed the best overall, with a 15% error rate for white speakers and 27% for black speakers. Apple performed the worst, with a 45% error rate for black speakers and 23% for white speakers.
So if you are black and you know the blood-boiling frustration of having to repeat information over and over again because the robot voice on the other line can’t seem to understand you no matter how clearly you’re speaking, now you know what the issue may very well be.
“This is yet another example of sampling bias that demonstrates the discriminatory impact on certain communities,” AI expert Sandra Wachter told Business Insider. “Compared to ‘traditional’ forms of discrimination ... automated discrimination is more abstract and unintuitive, subtle, intangible, and difficult to detect.”
“This type of bias testing is essential,” Wacher continued. “If we do not act now, we will not only exacerbate existing inequalities in our society but also make them less detectable.”
According to researchers, the issue is that automated systems learn by analyzing vast amounts of data. Just like facial recognition systems often make errors in recognizing people with darker skin because their databases are predominantly white, the data that voice recognition software learns from lacks diversity.
Basically, you’re shouting at Alexa at the top of your lungs because tech companies haven’t yet figured out that black voices matter.
“Here are probably the five biggest companies doing speech recognition, and they are all making the same kind of mistake,” John Rickford, one of the researchers for the study told behind the New York Times. “The assumption is that all ethnic groups are well represented by these companies. But they are not.”
People of color experience all kinds of bias, both implicitly and explicitly, in our daily lives. Who knew that something as simple as paying bills over the phone or shouting at Siri from across the room to play our favorite songs were among the myriad of things that prove more difficult for black people.
Hopefully, the CEOs of these big tech companies are paying attention to the studies telling them that they’re doing it wrong and correct these oversights as soon as possible.
I just want to pay my bills without giving myself a headache. That’s all.